How hearing aids address the problem of speech-in-noise in noisy and quieter places. We’ll also discuss what machine learning techniques are often used for noise reduction, and some promising strategies for hearing aids.
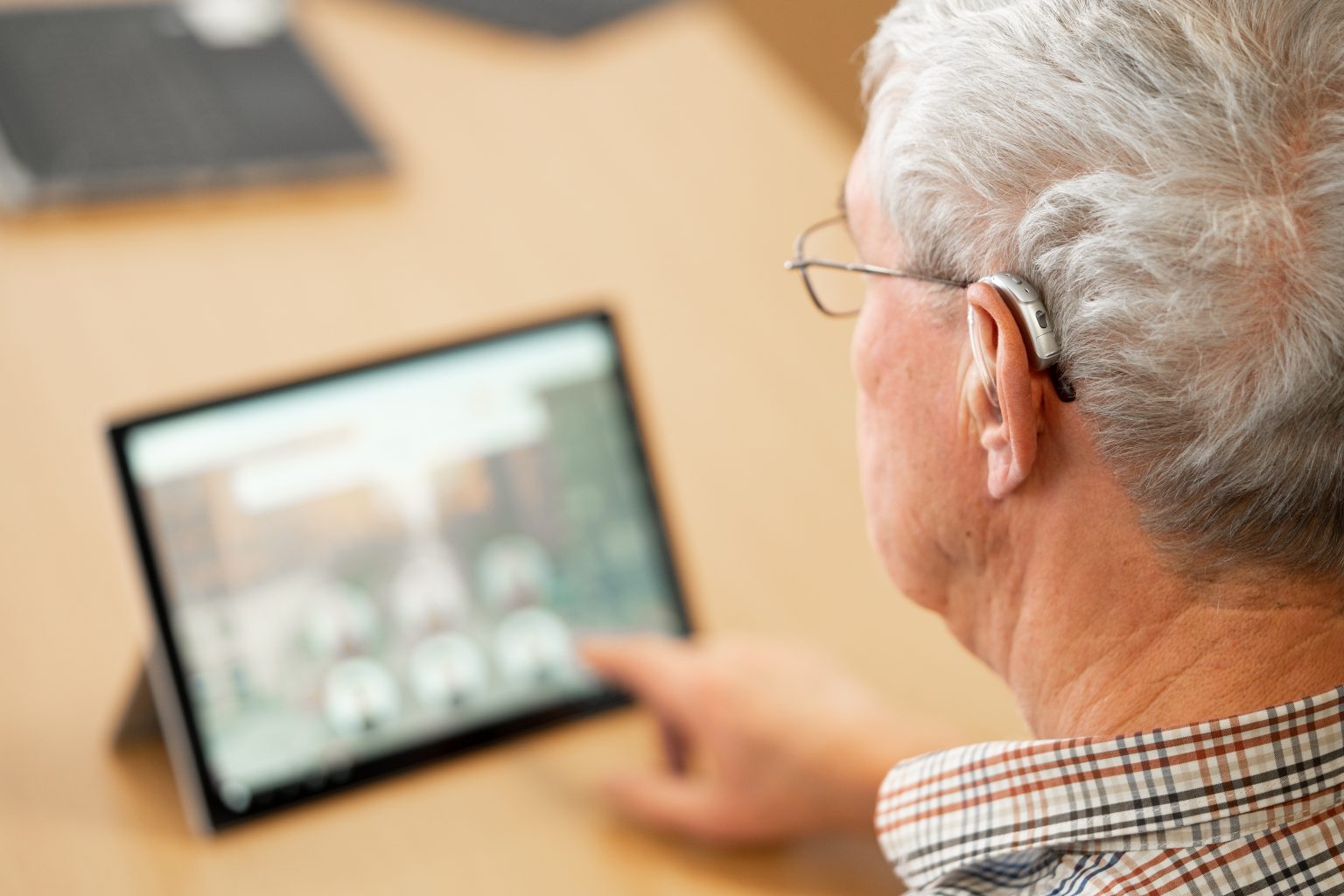
In a previous blog, we set out the problem of using hearing aids to pick out speech in noisy places. When the signal-to-noise ratio (SNR) is low, hearing aids can only do so much to improve the intelligibility of the speech.
A solitary hearing aid has various ways of addressing everyday constant noises such as cars, vacuum cleaners and fans. The aids work best when the noise is not too intrusive and SNR is relatively high. Problems arise when the noise is high (low SNRs), because then the hearing aid processing can distort the sound too much. While the hearing aid might have limited success in improving intelligibility in certain cases, they can still make the noise less annoying (e.g., Brons et al., 2014).
Using multiple microphones on each hearing aid can help in noisy conditions. The sound from the microphones is combined in a way that boosts the speech relative to the noise. This technology can be put into larger hearing aids, when there is enough spacing between the front and rear microphones.
One of the reasons why our brains are really good at picking out speech from the hubbub of a restaurant, is that it compares and contrasts the sounds from both ears. Our hearing is binaural. Similarly, if you have a hearing aids in both ears, they work better if they collaborate on reducing the noise.
Crucial to how our brains locate sound and pick out speech in noise are timing and level cues that come from comparing the sound at both ears. When sound comes from the side:
- interaural time differences occur because the sound arrives at one ear earlier than the other.
- interaural level differences occur because the sound has to bend around the head to reach the furthest ear.
Binaural hearing aids communicate wirelessly and use noise reduction strategies that preserve these interaural time and level difference cues (e.g., Van den Bogaert et al., 2009). This allows the listener’s brain to better locate the speech and boost this compared to the noise.